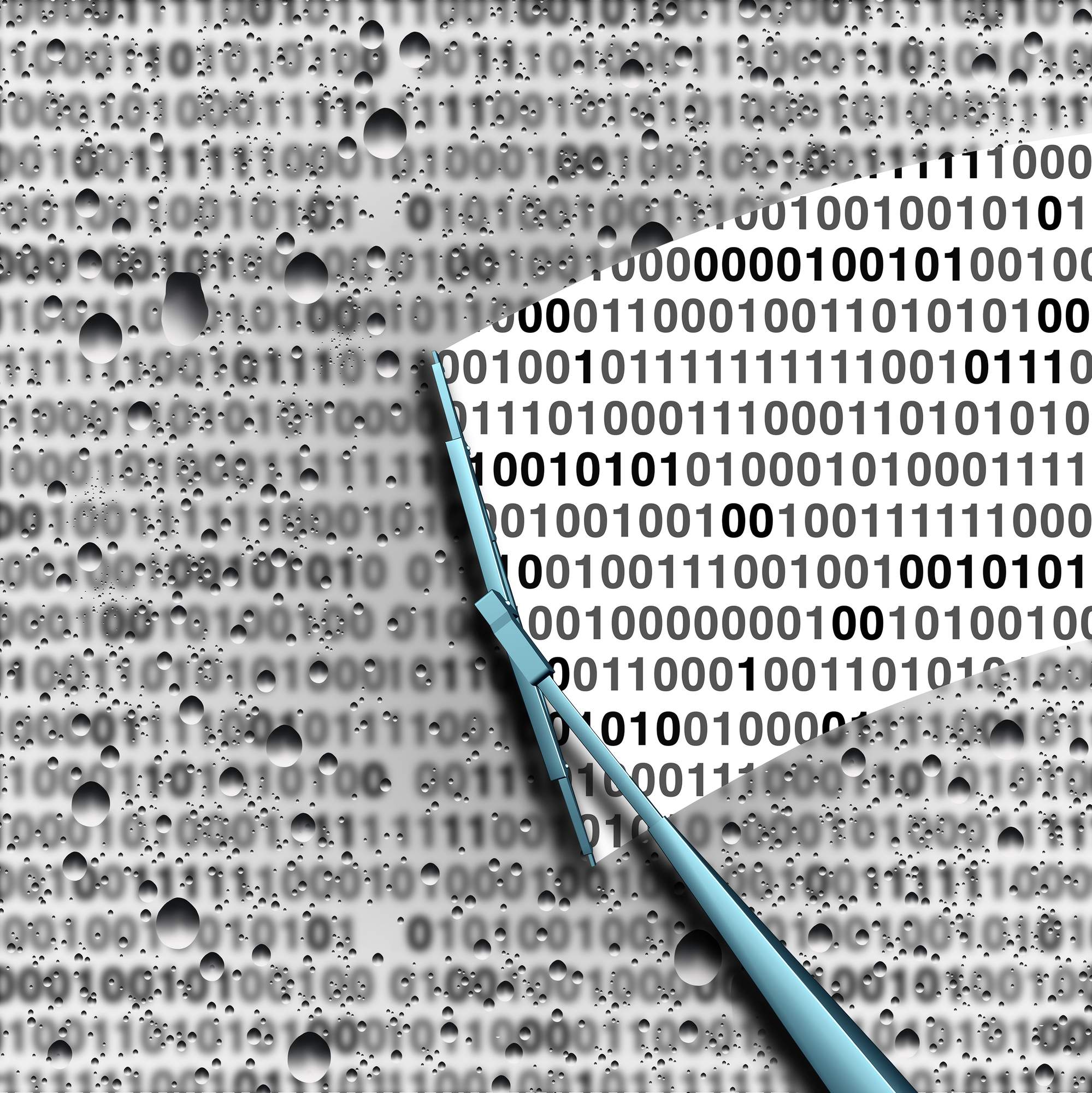
Blog / 4 Steps to Cleaner Data
Data cleansing is an important part of good data hygiene.
Because it can inform so many important business and strategic decisions, it’s essential for businesses and other organizations to maintain complete and valid data sets for whatever information you routinely collect and analyze. A single piece of data will often inform multiple decisions, such as both targeting a demographic for marketing and analyzing one in a focus group. And while a single piece of data alone is unlikely to throw off trends in truly enormous data sets, multiple errors can easily compound. That’s why it’s important to include data cleansing as both the first stage and as a regular part of an ongoing data maintenance program.
- Delete duplicates and other irrelevant data
- Fix formatting and other structural issues
- Fill in or discard incomplete data
- Locate and discard outliers
Delete duplicates and other irrelevant data
Duplicate data often occurs during collection and combining of data sets. You’re collecting data on subscribers and customers when they sign up for a service or to purchase a product. But when they forget their login credentials, can’t be bothered to reset them, or for some other reason decide to just make a new profile, well, that’s duplicate data. And if both duplicates get combined into a data then the errors follow your data around. In fact, duplicated data is likely the largest issue you’ll encounter in your data cleansing process, because there are plenty more ways it can happen.
That doesn’t mean there aren’t others types of data you need to cleanse, though. Irrelevant data are those that don’t relate to a given problem or question being analyzed. If your focus group targets Millenials, there’s no reason data for other age ranges should exist in a set. Removing these irrelevant data points, at least from specific sets, will make for more manageable and informative data.
Fix formatting and other structural issues
Deduplicating and discarding irrelevant data aren’t all there is to data cleansing. Throughout the process you’re likely to encounter inconsistencies in the way filenames are formatted. Typos, inconsistent capitalization conventions, and misspelled or improperly named files will all contribute to mislabeled data classes and categories. Acronyms can be particularly problematic in this area. For example, N/A and Not Applicable (and sometimes even things like TBD) may appear in your set, but should all be analyzed the same, so make sure your files are labelled correctly and consistently.
Fill in or discard incomplete data
Another issue to deal with during data cleansing will be incomplete data. Many algorithms and analytical tools won’t accept null values. Fortunately there are a few ways around this.
- Use other observations to find approximate or average values. Obviously you won’t be working from actual observations so there’s no getting around some data integrity loss, but it can be beneficial in some situations.
- In other situations, you may have to simply drop incomplete observations. This doesn’t just reduce data integrity but actually destroys some information in the process, so make sure you’ve made a considered decision before choosing this option.
Verify and discard outliers
One of the great things about data outliers is that they’re often easy to locate. There’s a reason they’re called outliers, after all; they sit outside the set of data like a sore thumb. Another is that most outlying data points are easily explained (many are often simple data entry errors) and can usually be deleted. That doesn’t mean you should go around erasing every outlier without hesitation though. Be sure to verify that the outlier really can be ignored. Just because a data point is an outlier doesn’t mean it’s invalid, and they can even sometimes prove or legitimize a hypothesis, so make sure you’re verifying the uselessness of an outlier before rightly removing it.
Although it often sounds like a large amount of work, data cleansing in only problematically time consuming if you allow your data hygiene practices to lapse and it severely decays (or if you’re just cleaning your data for the first time). Working regular (typically quarterly) data cleansing is an important step towards exceptional data management. If you’d like help developing a regular data cleansing procedure, contact a TRINUS account manager and find out why stress-free IT is the best IT.
Sincerely,
The TRINUS Team
trinustech.com